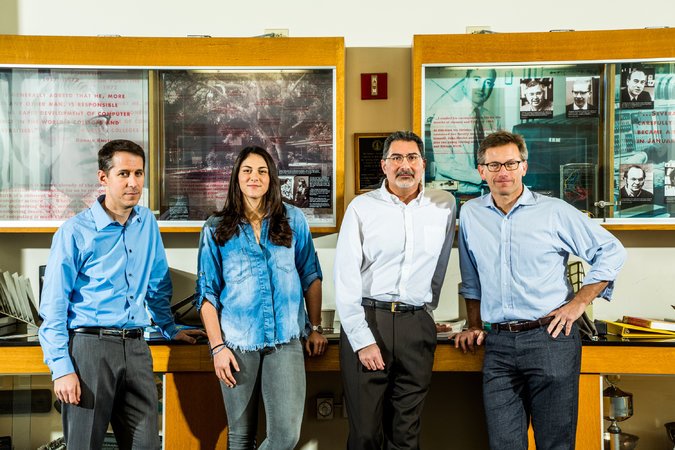
Jeremy Weinstein, Hilary Cohen, Mehran Sahami & Rob Reich of Stanford are developing a computer science ethics course for next year. (Photo Credit: Christie Hemm Klok for The New York Times)
In the wake of increasing concerns about artificial intelligence and the somewhat predominant “lack of ethical considerations” in the industry relative to its possible social ramifications, some universities are starting to bring a “more medicine-like morality to computer science.”
Rather than holding tight to the ethos “build first and ask for forgiveness later,” (a common, and unfortunate, modus operandi in the field), technologists — such as Jeremy Weinstein, Hilary Cohen, Mehran Sahami & Rob Reich of Stanford University — are pushing hard to establish a new ethos akin to that in the medical field: First and foremost, do no harm.
“Technology is not neutral,” said Professor Sahami, who formerly worked at Google as a senior research scientist. “The choices that get made in building technology then have social ramifications.”
The idea is to train the next generation of technologists and policymakers to consider the ramifications of innovations — like autonomous weapons or self-driving cars — before those products go on sale.
The new ethics course covers topics like artificial intelligence and autonomous machines; privacy and civil rights; and platforms like Facebook. Rob Reich, a Stanford political science professor who is helping to develop the course, said students would be asked to consider those topics from the point of view of software engineers, product designers and policymakers. Students will also be assigned to translate ideal solutions into computer code.
Self-Taught AI & The Real World
When considering ethical implications, one must also look at where things are at currently relative to smart technology, and how a particular technologies’ “genetic make-up” (e.g., the petri-dish from which a machine operates) might translate to real-world scenarios (scenarios outside the petri-dish?) and help us assess possible and probable outcomes (and the moral considerations that obtain).
“Research teams like DeepMind hope to apply [game-theory] methods to real-world problems like building room-temperature superconductors, or understanding the origami needed to fold proteins into potent drug molecules. And of course, many practitioners hope to eventually build up to artificial general intelligence, an ill-defined but captivating goal in which a machine could think like a person, with the versatility to attack many different kinds of problems.”
According to some researchers, however, it isn’t yet clear how far past the game board the current techniques can go. Why?
From an algorithm’s perspective, problems need to have an “objective function,” a goal to be sought. When AlphaZero played chess, this wasn’t so hard. A loss counted as minus one, a draw was zero, and a win was plus one. AlphaZero’s objective function was to maximize its score. The objective function of a poker bot is just as simple: Win lots of money.
Real-life situations are not so straightforward. For example, a self-driving car needs a more nuanced objective function, something akin to the kind of careful phrasing you’d use to explain a wish to a genie. For example: Promptly deliver your passenger to the correct location, obeying all laws and appropriately weighing the value of human life in dangerous and uncertain situations. How researchers craft the objective function, [Computer Scientist, Pedro] Domingos said, “is one of the things that distinguishes a great machine-learning researcher from an average one.”
But this reveals a deeper issue. How great is our understanding of the world as humans? One might claim that yet another challenge for translating and applying “machine-think” to “human think” has to do with the structure of the real world itself and our (while impressive) still fragile epistemic hold of its more “mysterious” inner-workings. In other words, capturing all of the variables and subtle nuances of the the things that constitute reality is no simple task, especially when our grasp of these things is tenuous at best.
“There is a huge difference between a true perfect model of the environment and a learned estimated one, especially when that reality is complex,” wrote Yoshua Bengio, a pioneer of deep learning at the University of Montreal, in an e-mail.
When it comes to creating and using smart technology in real-world scenarios, says Chelsea Finn, a Berkeley doctoral student who uses AI to control robot arms and interpret data form sensors: “for self-play systems to produce helpful data, they need a realistic place to play in.“
“All these games, all of these results, have been in settings where you can perfectly simulate the world,”…Other domains are not so easy to mock up. Self-driving cars, for example, have a hard time dealing with bad weather, or cyclists. Or they might not capture bizarre possibilities that turn up in real data, like a bird that happens to fly directly toward the car’s camera. For robot arms, Finn said, initial simulations provide basic physics, allowing the arm to at least learn how to learn. But they fail to capture the details involved in touching surfaces, which means that tasks like screwing on a bottle cap — or conducting an intricate surgical procedure — require real-world experience, too.
For problems that are hard to simulate, then, self-play might not be so useful. This seems to suggest that researchers interested in assessing possible ethical implications of artificial intelligence (before they are produced) have an even harder task ahead. It is difficult enough to imagine how things might play out and what might be at stake in everyday life as human players in the world (employing a new means of technology). But what about imagining a new sort of player altogether? And moreover, a new player making “real-world decisions” based on data from simulations that are (quite probably) lacking in some sense or another?
Challenge aside…the task at hand is a worthy one…and something for which we are glad to see researchers starting to take seriously. Read this article in its entirety here!
**NOTE: For more on this subject, see also Part 1, Part 2 & Part 3 of our 2016-2017 Philosophy Symposium series “Vulnerable Humanity, Predictable Machines.”**